Machine Learning and Audit
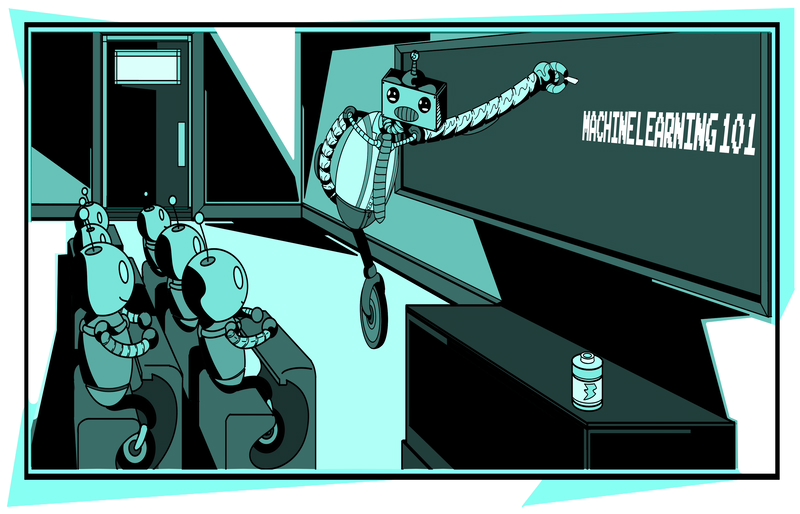
Machine Learning and Audit
The moment you talk about Machine Learning, the world drifts to a mythical zone. A place of futuristic predictions with data and numbers. But that is only partially true.
Machine learning harnesses the power of algorithms to derive predictions from data. Computational machines learn and improve themselves in this process to predict better. Hence Machine Learning.
An example of machine learning is the price prediction by a ridesharing app. The surge or fall in the price for the same route is because of ML that calculates the traffic and cost. Next time when you give a few lesser bucks than usual on the same route, thank ML.
Benefits of Machine Learning for Businesses
Cognitive technologies like ML are proving to be effective in the marketing and sales domains. Want to predict customers’ behavior or generate leads? A well-built ML model with the right data is your go.
Collected data can generate actionable business insights for making business decisions.
ML algorithms can deduce the customer’s pain points. AI-driven chatbots can direct them to the right team if unable to answer (the chatbot on this site is managed by an actual person so feel free to ask away!). You can speed the service and improve the products based on the insights. Thus, sales and marketing can achieve greater heights.
ML can help harness hidden operational efficiency. ML programs can infer upcoming breakdowns and probable accidents in the factories nowadays.
Risks Machine Learning Poses to Businesses
While it all appears utopian with ML, many pitfalls can make ML algorithms fail miserably.
One such blooper was the model predicting crime based on people’s history. It not only put a juvenile on a higher side of crime prediction than a seasoned robber but also behaved racist. Imagine, a machine was racist!
No wonder even a small glitch can drive ML models to pose risks to a business and vilify them.
- Biases in Input Data
ML algorithms rely heavily on input data. Insufficient, outdated, limited, or wrong data can influence the outputs of the model. Sometimes, training data fed to the algorithm fluctuate so much from actual data that the results vary drastically.
Incorrect data collection methods lead to wrong predictions, too.
- Misused Data and Ethical Issues
Facebook blocked a UK based insurer in 2016 who exploited users’ data. The offender offered insurance to first-time drivers based on their driving skills. This was a clear risk to the ethical use of data.
Ethics is a question of debate in ML because when we question ethics, we question humans.
Ethics—as one can understand—is very difficult to imbibe tangibly in a system. ML is no different.
- Security Risks
Adversarial inputs and malicious attacks are getting rampant. Attempts to hack users’ data—the core of ML—are increasing too. Misguiding the model with manipulated data to generate skew the outcome is not any less.
Tampering a biometric recognition system could be an example.
- Incorrect Interpretations
A small misinterpretation can lead to blunders. If the assumptions are inapt or incomplete, the model might miss a few parameters. This can land you with deviated outputs.
The ever-growing complexity in the models is making the algorithms a black box. Hence, sometimes, the developers—although cautious about known biases—are clueless of results.
Risk Mitigation in Machine Learning
- Authenticated and Certified Data
Tampering data to avoid the truth or produce a favorable outcome needs elimination at the source. Data from authenticated systems should be used while training the models. Tagging data for source identification could be one way. The market has tools available that can certify data reliability.
- Root Out Biases
Data collection should happen on a broader level. The narrow focus should be widened to accommodate the bigger population and events. Including a diverse range of opinions, events, and populations can help cut the biases. Training data should be as close as actual data.
- Transparency and Interpretability
ML models are usually proprietary of the owners and are highly complex and opaque. These make them tough to audit holistically. Companies should keep a certain level of transparency in the algorithms. This will ease the audit pains.
- Regulation, Compliance, and Governance
The mitigation associated with ML risks is different from the traditional perils. The reasons are the opaque algorithms lending unpredictability to the programs. These proprietary models need specially designed standards and regulations.
Developers usually focus on precisely training the model. When it comes to reality, governance goes haywire. Companies should adopt a rounded regulatory approach. Additionally, they should conjoin auditing with ML projects for risk mitigation efficacy.
Before We Leave
If your audit department isn’t using ML then talk to us to help you become a leader in implementing tech in the audit industry.